- Karina Datascientist's Newsletter
- Posts
- How Recommendation Engines and Generative AI Are Changing Customer Experience
How Recommendation Engines and Generative AI Are Changing Customer Experience
More and more companies today are fighting for customer's attention. We all have endless amount of apps installed, so what is the way to capture customer's attention? One of the option is personalisation - creating content, feed, features relevant to each and every specific customer. It is like buying a tailored made suit vs fast fashion suit. Both are suits, but one just fits perfectly.
In today's newsletter we will talk about personalisation done via recommendation engines (machine learning) and generative AI.
Netflix suggests you the movie you might be interested in, Spotify knows what song to play, Amazon suggests what other product you might want to buy - all of these are examples of recommendation engines.
These sophisticated systems analyse your past behaviours, preferences and interactions to predict what you might enjoy next. But how exactly do they work?
The Core Methods Behind Recommendation Engines
Collaborative Filtering: This approach relies on patterns of the crowd, thus the name. It assumes that if Person A and Person B have similar tastes in certain items, they might have similar preferences for other items too. For example, if you and another user both rated the same five movies similarly, Netflix might recommend you films that this other user enjoyed but you haven't watched yet. When an ML model is created using this approach, it is always important to ask a first-time customer about their preferences in order for recommendations to work. That's why when you sign up to Netflix for the first time, they ask you to select a couple of favourite movies.
Content-Based Filtering: This method focuses on the attributes of products and your demonstrated preferences. This is more about you and less about the crowd. If you frequently read articles about artificial intelligence, a content-based system will recommend more AI-related content, regardless of what other users are doing.
Hybrid Approaches: Most modern systems combine both methods, creating recommendations based on both your personal preferences and patterns observed among similar users.
Examples
Spotify's Discover Weekly: Creates a personalised playlist by analysing your listening habits and finding songs with similar audio attributes to ones you enjoy, as well as songs enjoyed by users with similar tastes.
Amazon's "Customers who bought this also bought": Leverages the collective purchasing behaviour of millions of customers to suggest products you might be interested in.
My favourite example of a recommendation engine gone wrong is the below image:
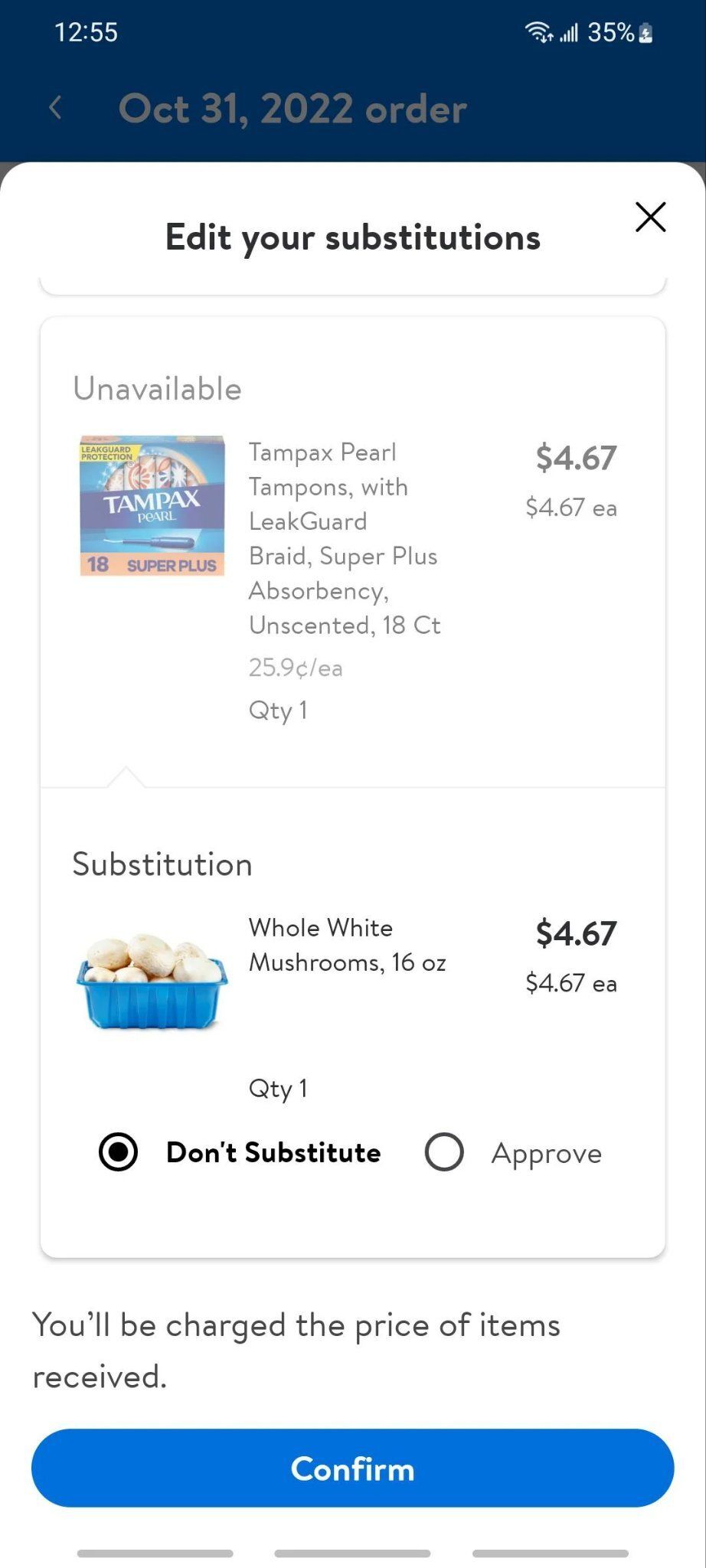
Walmart suggested mushrooms as an alternative to a sanitary item (same price, both are white). That is definitely not an equivalent replacement.
Recommendation engines used for product substitution are usually a bit different from Netflix/Spotify. They are more rule-based, as you need to make sure that the item is in stock, preferably same brand, size, category and around the same price; however, they do have ML add-ons where, based on the acceptance of other users, they might decide if a mushroom is the right choice for someone who has periods (spoiler alert: it's not).
The Rise of Generative AI in Personalization
While recommendation engines work with selecting existing content, generative AI can create entirely new, custom content for individual users.
How Generative AI Works
Generative AI systems like GPT (Generative Pre-trained Transformer) models are trained on vast amounts of data and learn patterns in language, images, other media. They can then generate new content that follows these patterns. For personalisation, these systems can:
Learn from user interactions: By analysing how you communicate, what topics you engage with and your preferences, generative AI can adapt its outputs to match your style and interests.
Create on-demand content: Rather than simply recommending existing content, these systems can generate new material specifically tailored to your needs and preferences.
Adapt to context: Modern generative AI can understand the context of your requests and generate appropriate responses that take into account your history and current situation.
Applications
Personalised Email Marketing: Beyond just inserting your name, AI can now generate entirely unique email content based on your purchase history, browsing behaviour and engagement patterns.
Individualised Learning Materials: Educational platforms can generate explanations, examples, and practice problems that adapt to each student's learning style and current understanding.(Duolingo)
The Power of Combining Both Approaches
The most powerful personalisation systems today combine recommendation engines with generative AI:
Recommendation engines identify what content or products might interest you.
Generative AI customises how that content is presented to maximise relevance and engagement.
For instance, a news app might use a recommendation engine to select topics of interest to you, then employ generative AI to create a summary in your preferred reading style and level of detail.
Conclusion
Like that perfectly tailored suit, well-executed personalisation simply feels right – not intrusive, but attentive to individual needs and preferences.
And based on industry trends, these skills are in high demand, as more and more businesses are fighting for customers' attention. So if you like AI, ML and data - it might be worth spending more time learning about personalisation.
Keep pushing 💪,
Karina
ChatGPT images
ChatGPT updated their image models, and the results are phenomenal. You might have seen the results of personal images converted into a style of Studio Ghibli. And now there is a new trend - converting your pet photo into a realistic human and vice versa.
Here is a photo of seal 🦭 Arthur and the human equivalent. Very impressive.
Prompt you can use:
"Convert this animal into a realistic human version. Use its physical features (like ears, eyes, fur/colour, posture, and size) as inspiration for the human's hairstyle, facial features, clothing, and expression. The result should be a realistic photograph of a human (male/female), without any animal parts. Maintain the essence and personality of the animal in the human character."
![]() | ![]() |
Need more help?
Just starting with Python? Wondering if programming is for you?
Master key data analysis tasks like cleaning, filtering, pivot and grouping data using Pandas, and learn how to present your insights visually with Matplotlib with ‘Data Analysis with Python’ masterclass.
![]() | Data Analyst & Data Scientist |